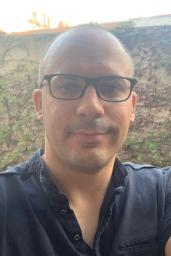
Marc Plantevit
Professor
Artificial Intelligence
The AI Team is a newly created team at LRE. Its scientific activity is devoted to Knowledge Discovery from Data using automatic or semi-automatic techniques. This includes data mining, machine learning, speech processing, statistical learning, deep learning, data analysis, exploratory data analysis. Its research interests include data mining, speech processing and explainable AI.
The team leverages about 4 permanent researchers whose computer skills and research interests are complementary. They are brought together by the aim to produce added value from data (I.e., models, useful patterns or knowledge).
More specifically, the team is working on the following problems:
Our results are theoretical, methodological, algorithmic, software, and applications. Our guiding principle is to try to help data owners throughout the interactive process of knowledge discovery from data. Such processes require the combination of a wide range of paradigms of description or induction (pattern extraction, classification, statistical learning, deep learning, etc.). Applications
Our research is developed in relation to real data analysis: the quantitative and qualitative empirical study on real data is fundamental to assessing the performance of the proposed methods. While AI research team develops methods and algorithms rather than applications, it works with owners of data from several environments: Neuroscience, Chemistry, Social Sciences, Industry, etc. This diversity shows our willingness to be centered “Methods” and develop generic algorithms applicable to a broad spectrum of applications.
Our research is developed in relation to real data analysis: the quantitative and qualitative empirical study on real data is fundamental to assessing the performance of the proposed methods. While AI research team develops methods and algorithms rather than applications, it works with owners of data from several environments: Neuroscience, Chemistry, Social Sciences, Industry, etc. This diversity shows our willingness to be centered “Methods” and develop generic algorithms applicable to a broad spectrum of applications.
The major annual conferences are: ECMLPKDD, SIGKDD, IEEE ICDM, SIAM DM, PAKDD, DSAA, IJCAI, AAAI, Neurips, ICML, ICRL,WWW, RecSys, WSDM, VLDB, EDBT, ICASSP, INTERSPEECH, Odyssey, SLT.
The major journals are: Journal of Machine Learning Research, Articificial Intelligence Journal, Pattern Recognition, Machine Learning, Neurocomputing, Data Mining and Knowledge Discovery, KAIS, IEEE TASLP, and Speech Communication.
Significant contributions can also occur in conferences (and journals) of data management (e.g., VLDB, ACM CIKM, IEEE TKDE, Information Systems).
xAI
Explainable AI (xAI) research is crucial for the AI scientific community as it bridges the gap between complex AI models and human understanding, fostering trust, transparency, and accountability. By making AI decisions interpretable, xAI enhances the reproducibility and reliability of scientific findings, addresses ethical concerns, and ensures compliance with regulatory standards. This not only accelerates the adoption of AI in critical fields such as healthcare, finance, and environmental science but also empowers researchers to identify and mitigate biases, ultimately leading to more robust and fair AI systems.
Marc Plantevit
Professor
Idir Benouaret
Associate Professor
Réda Dehak
Associate Professor
Laurence Denneulin
Associate Professor
Lamine Diop
Associate Professor
Julien Perez
Associate Professor
Théo Lepage
PhD Student